Armoured Fighting Vehicles (AFVs) are military vehicles designed for combat, combining armor protection, firepower, and mobility. Examples include tanks, infantry fighting vehicles, and armored personnel carriers.
They operate in extreme environments—rough terrain, temperature fluctuations, and combat stresses—which demand rugged mechanical systems like tracks, heavy engines, and complex weaponry.
Challenges of Reactive Maintenance Strategies
Armoured Fighting Vehicles (AFVs) are challenging to monitor visually due to their complexity - of structure, components and systems. Traditional maintenance strategies rely on manual data collection or predefined schedules. This can lead to
- Premature equipment failure and reduced vehicle lifespan.
- Frequent downtime, impacting mission readiness.
- Escalating costs due to unplanned corrective maintenance.
While AI based predictive maintenance are being used for real-time health monitoring of AFVs, the complexity and mission readiness necessitates a solution that leverages advanced analytics to optimize maintenance schedules and enhance operational efficiency.
BQP’s Solution:
BQP’s quantum powered platform BQPhy®, utilizing its Quantum Enhanced Machine Learning (QML) solver employs a hybrid quantum neural network, combining classical and quantum computing strengths to accelerate transfer learning in big data with accuracy levels potentially surpassing classical machine learning. The solution addresses the problem by:
- Collecting vast amounts of data from AFV sensors.
- Encoding this data into quantum states via quantum feature mapping for efficient processing.
- Using quantum algorithms for pattern recognition to identify complex correlations that classical computers might miss.
- Generating predictions for potential failures or maintenance needs based on analyzed patterns.
- Optimizing maintenance schedules and resource allocation using quantum algorithms.
- Continuously learning and updating the model with new data to improve predictions over time.
Tangible Outcomes of Quantum-Powered Maintenance*
1. Reduce Maintenance Costs by 30–40%
By shifting from reactive to predictive strategies, BQP’s solution helps reduce unplanned breakdowns and optimizes maintenance cycles—cutting down on unnecessary spending and resource wastage.
2. Increase AFV Availability by Up to 20%
Continuous monitoring and predictive fault detection minimize downtime, ensuring that more AFVs are operational and ready for deployment during mission-critical moments.
3. Extend Equipment Lifespan by 15–20%
Early identification of potential failures helps prevent long-term damage, significantly extending the service life of subsystems and mechanical components.
4. Ensure Real-Time Mission Readiness
Quantum-powered diagnostics provide real-time insights during operations, making sure AFVs remain functional and combat-ready in high-pressure environments.
5. Scale with Future Defence Needs
BQP’s solution is built to support system upgrades and integrations, aligning with India’s long-term defence modernization goals and evolving battlefield requirements.
*Estimates only for representative purposes
BQP’s breakthrough bridges the gap between theoretical quantum advantages and practical, deployable solutions—without requiring quantum hardware.
How BQP’s Solution Addresses the Problem
BQP’s Quantum-Powered AFV Health Monitoring System combines quantum computing and machine learning to deliver superior predictive maintenance:
Research, such as Quantum for Transportation & Mobility - Pasqal, indicates that quantum computing excels in predictive analytics for fleet management, predicting breakdowns more accurately and minimizing downtime, which is directly applicable to AFVs. Additionally, [Quantum Computing for Predictive Analytics Real-World
Applications (https://quantumzeitgeist.com/quantum-computing-for-predictive-analytics-real-world-applications/) highlights quantum algorithms’ potential for machine learning tasks like clustering, offering exponential speedup over classical methods, supporting BQP’s claims of enhanced accuracy and efficiency.
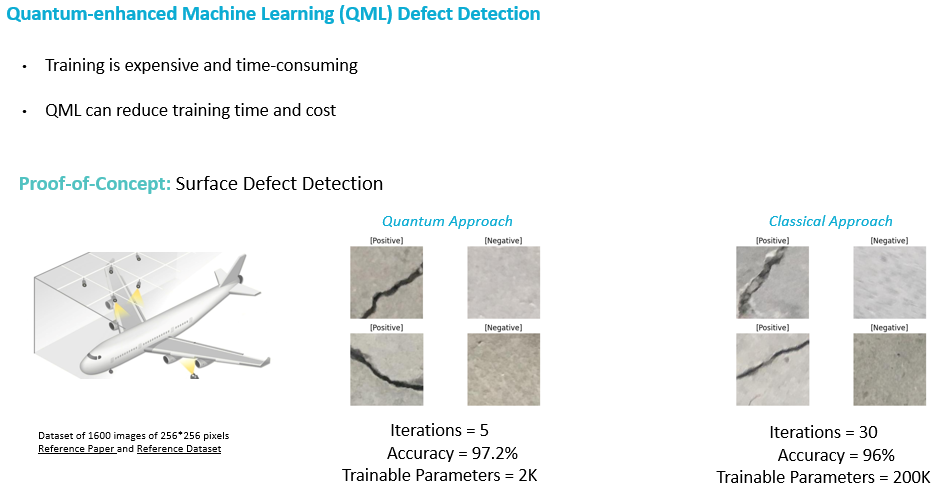
As can be seen from the above test for enhancing image processing for surface defect detection, BQPhy®’s Quantum Machine Learning achieves an accuracy of 97.2% with just 2K trainable parameters in 5 iterations. Classical method working on the same data set requires 200K trainable parameters, using 30 Iterations and achieving an accuracy of 96%
Several Use cases: Besides predictive maintainence, Quantum-Enhanced Machine Learning (QML) solvers have a wide range of defence applications and use cases and can significantly improve defect detection with fewer iterations and fewer parameters as compared to classical algorithms for machine learning